Robots have undergone a significant upgrade in their footballing capabilities, thanks to the application of deep reinforcement learning, a form of artificial intelligence. Guy Lever and his team at Google DeepMind have propelled two-legged robots, trained using this cutting-edge technique, to perform tasks such as walking, turning to kick a ball, and recovering from falls at a remarkable pace compared to their counterparts trained using scripted lessons.
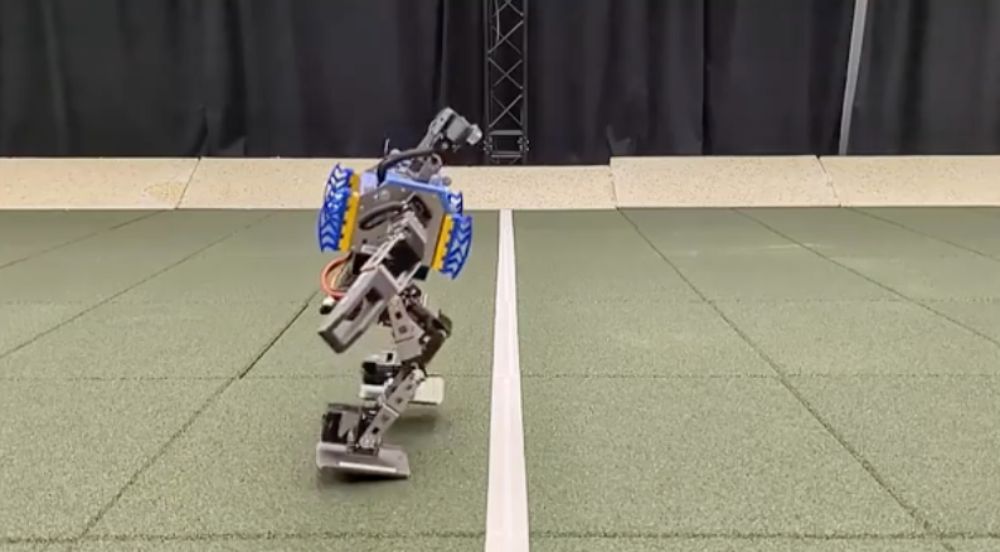
The robots, powered by battery and standing at approximately 50 centimeters tall with 20 joints, underwent an extensive training regimen of 240 hours using deep reinforcement learning. This method combines the principles of reinforcement learning, where agents learn through trial and error, and deep learning, which utilizes neural networks to analyze patterns within the data presented to the AI.
In comparison to robots trained with pre-scripted skills, those trained using deep reinforcement learning exhibited remarkable improvements. They could walk 181 percent faster, turn 302 percent quicker, kick a ball 34 percent harder, and recover from falls 63 percent faster in a one-on-one game. Lever notes, “These behaviors are very difficult to manually design and script.”
Jonathan Aitken, from the University of Sheffield, UK, highlights the significance of this research in advancing robotics, particularly in addressing the challenge of bridging the gap between simulated and real-world environments. Leveraging a physics engine to simulate training scenarios rather than relying solely on real-life trials proves to be an effective approach in ensuring the transferability of learned skills to practical applications.
However, Tuomas Haarnoja, a member of the Google DeepMind team, emphasizes that the ultimate goal is not to create football-playing humanoid robots for professional leagues. Instead, the focus lies in understanding how to rapidly develop complex robot skills using synthetic training methodologies that can be seamlessly and robustly applied to real-world scenarios.
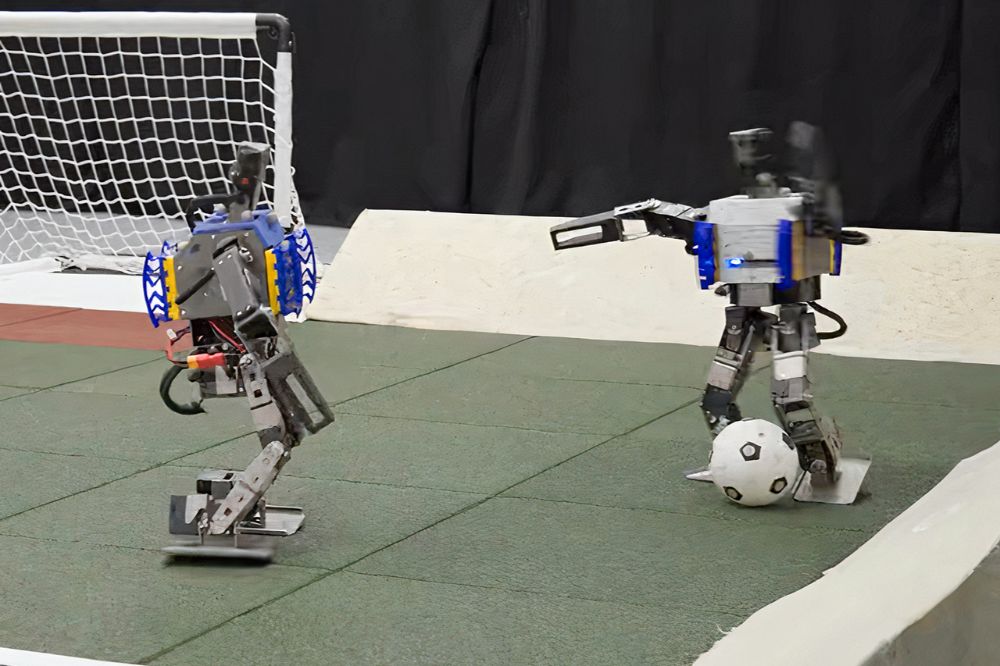
Aitken echoes this sentiment, emphasizing that while soccer-playing robots may not be the immediate aim, the research sheds light on the potential of synthetic training methods to facilitate the rapid and reliable development of skills applicable across various practical domains.
The advancements showcased in Lever and his team’s research underscore the transformative potential of deep reinforcement learning in revolutionizing robotics, paving the way for the rapid acquisition and deployment of sophisticated robot skills in real-world applications.