Elon Musk’s Optimus Tunic, which was developed by Tesla, has garnered attention on social media for its ability to pour coffee and pick up objects. However, some people incorrectly believe that these robots operate autonomously for all tasks. In reality, robots often operate through teleoperation systems, where human demonstrations play a crucial role. The robots operate through teleoperation systems. Skilled individuals, such as roboticists trained in a specific field and located at a certain distance, control humanoid robots’ actions. While the public may not always be aware of this, teleoperation is often the method through which humanoid robots are operated, not full autonomy.
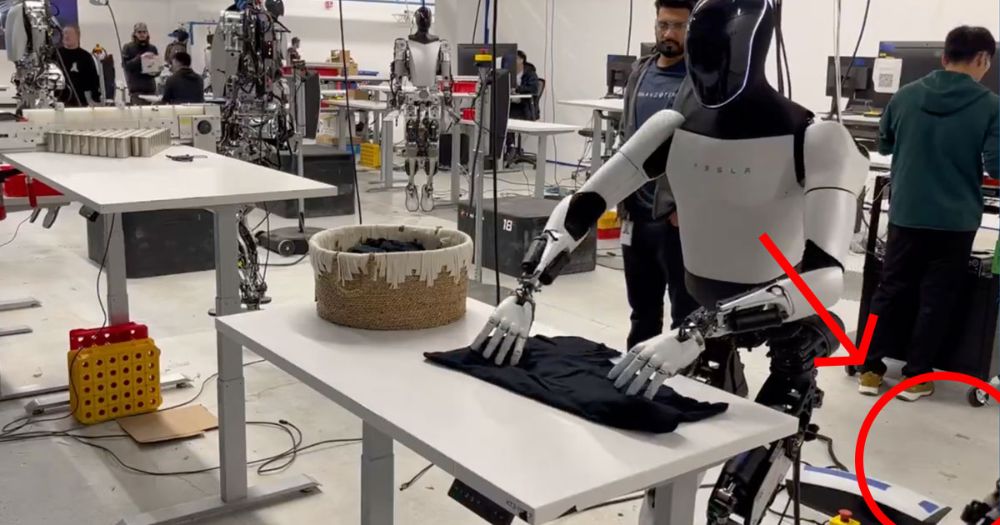
Companies like Boston Dynamics serve as excellent examples of this. All humanoid robots from Boston Dynamics are teleoperated. Additionally, when humanoid robots are seen in various media, it’s often assumed that they operate autonomously. However, it’s possible to determine whether a video features teleoperation or autonomy based on the speed of movement. Teleoperation implies a slower, more controlled movement, while autonomy typically involves faster movement. This distinction is essential, as teleoperation involves direct human control over humanoid robots, whether through teleoperation systems or other means like connecting to computers or cables.
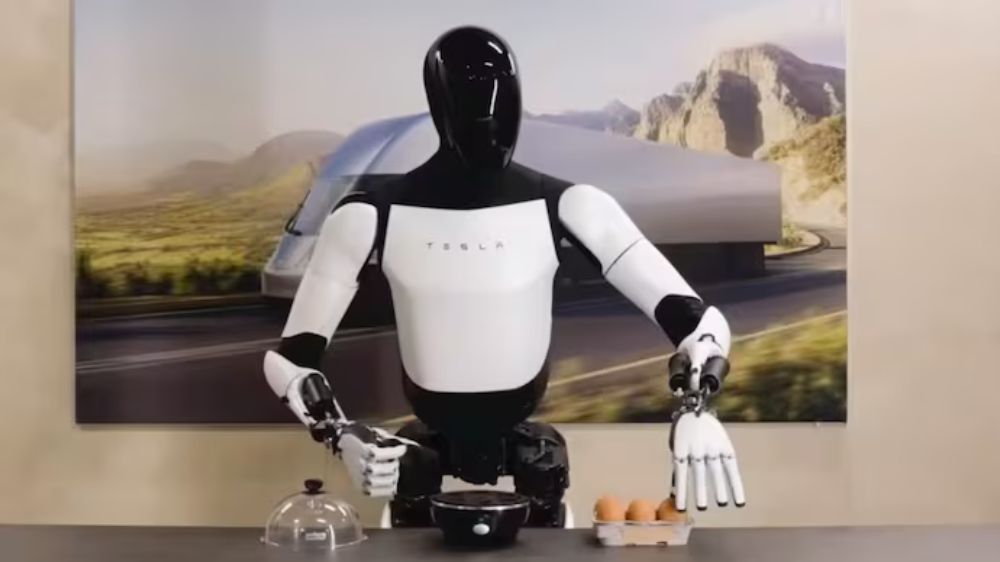
Recent advancements in imitation learning from human demonstrations have demonstrated impressive performance in robotics, particularly in table-top manipulation tasks. However, there’s a gap in applying these techniques to mobile manipulation tasks that require bimanual coordination and whole-body control.
To address this gap, researchers have developed Mobile ALOHA, a low-cost teleoperation system that integrates a mobile base and a whole-body teleoperation interface. By collecting data using Mobile ALOHA, researchers conducted supervised behaviour cloning and found that co-training with existing static ALOHA datasets significantly improved performance on mobile manipulation tasks.
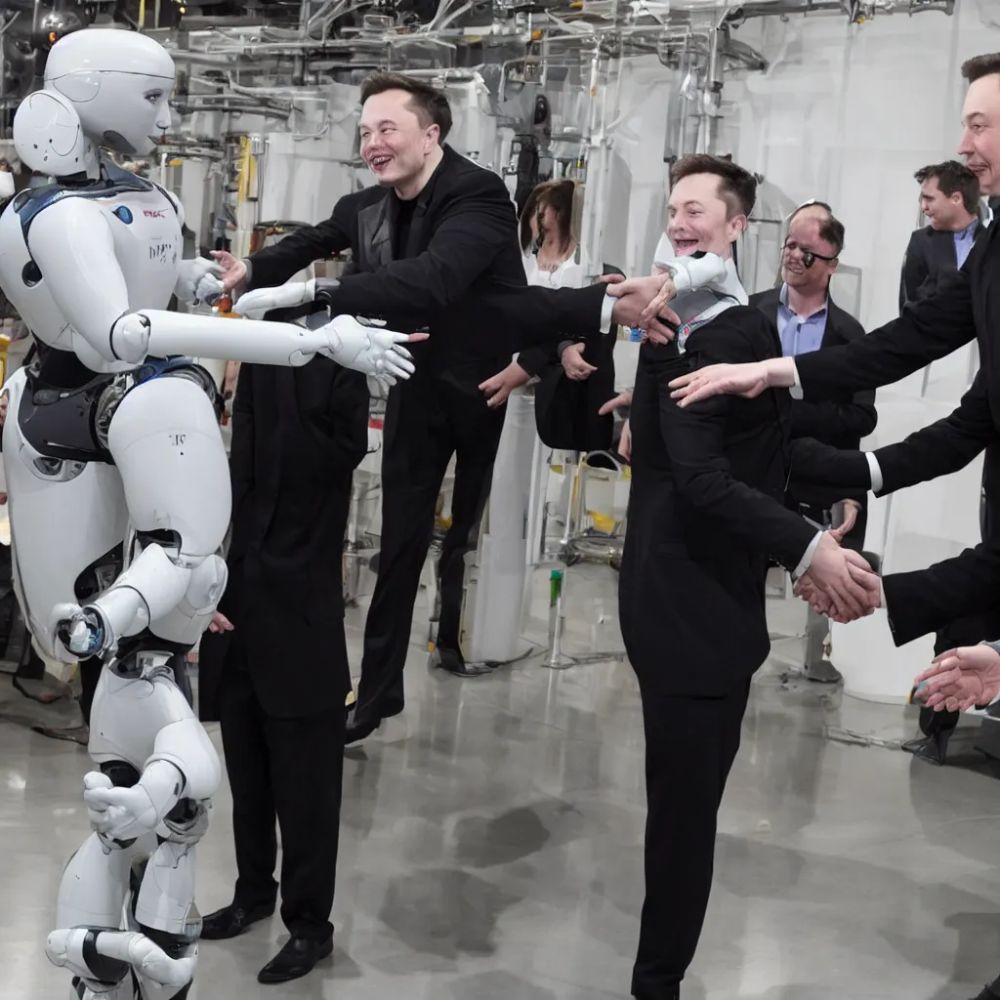
With just 50 demonstrations for each task, co-training increased success rates by up to 90%. This enabled Mobile ALOHA to autonomously perform complex mobile manipulation tasks such as cooking, storing heavy cooking pots, using an elevator, and rinsing a pan using a kitchen faucet.
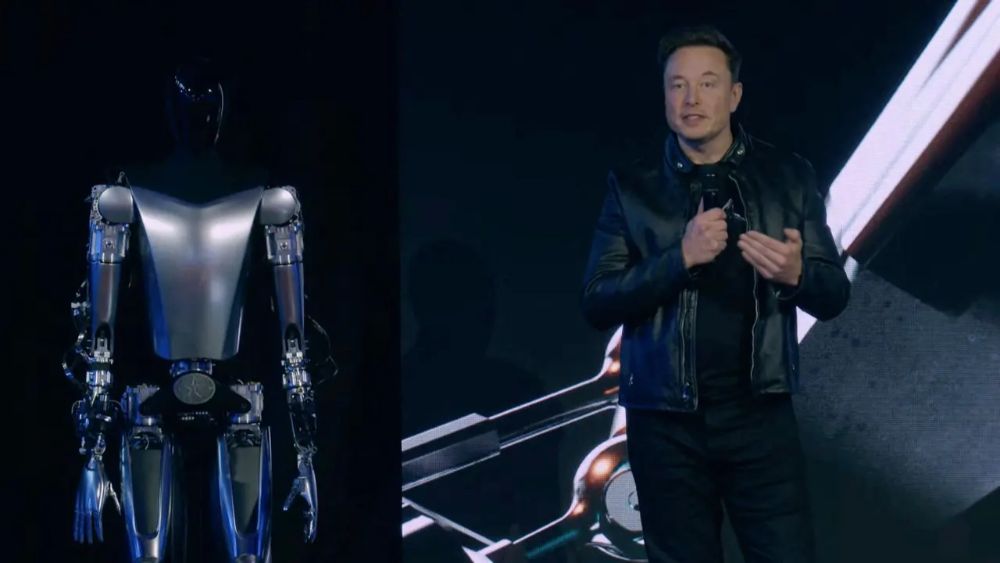
The robot is trained using both a database and supervised demonstrations. Demonstrations are conducted using a device attached to the robot, allowing it to mimic necessary actions for a given task. Training involves driving the robot around and demonstrating tasks, akin to puppetry.
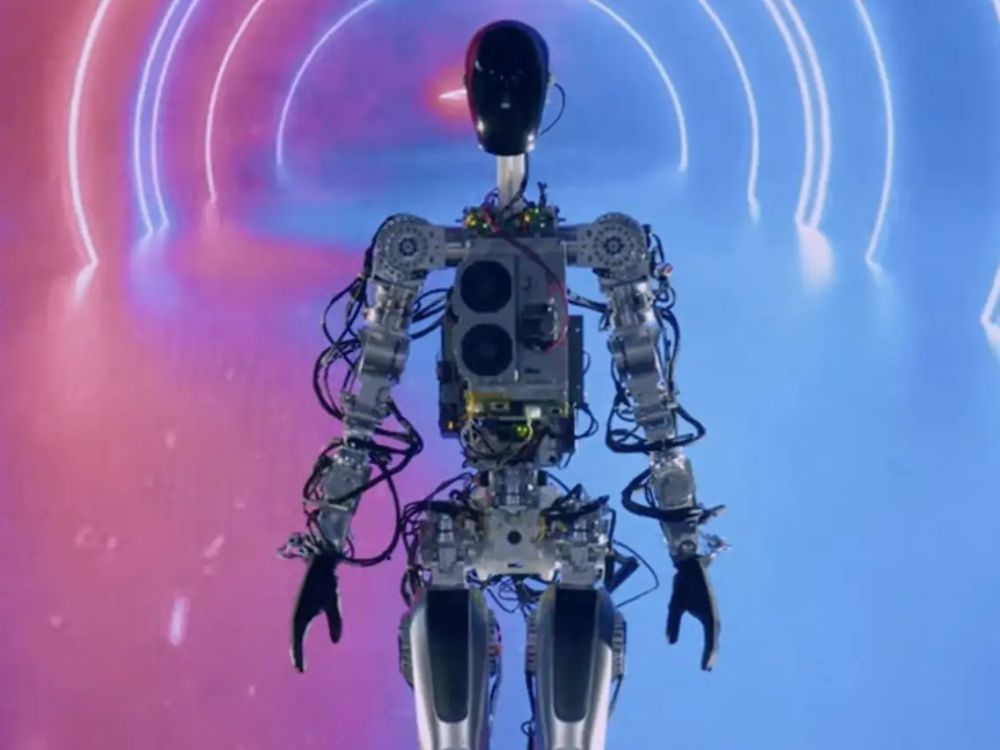
Mobile and untethered, the robot features a large battery in its base for extended work periods and stability. It autonomously performs various household tasks, from cooking to laundry, showcasing its versatility and capability in real-world scenarios. Videos of the robot in action highlight its ability to learn and execute a wide range of tasks, demonstrating the potential of teleoperation-based learning in robotics.