Many people across Southeast Asia have been exploring the use of large language models like Meta’s Llama 2 and Mistral AI, but they often do so in their native languages such as Bahasa Indonesia or Thai. However, the resulting output in English is typically nonsensical, which puts them at a disadvantage, according to tech experts. This comes as generative artificial intelligence is rapidly changing various aspects of life, including education, work, and governance worldwide. To address this issue, a Singapore government-led initiative has introduced SEA-LION (Southeast Asian Languages in One Network), the region’s first large language model trained on data from 11 Southeast Asian languages including Vietnamese, Thai, and Bahasa Indonesia. This open-sourced model is designed to be more cost-effective and efficient for businesses, governments, and academia in the region, according to Leslie Teo from AI Singapore.
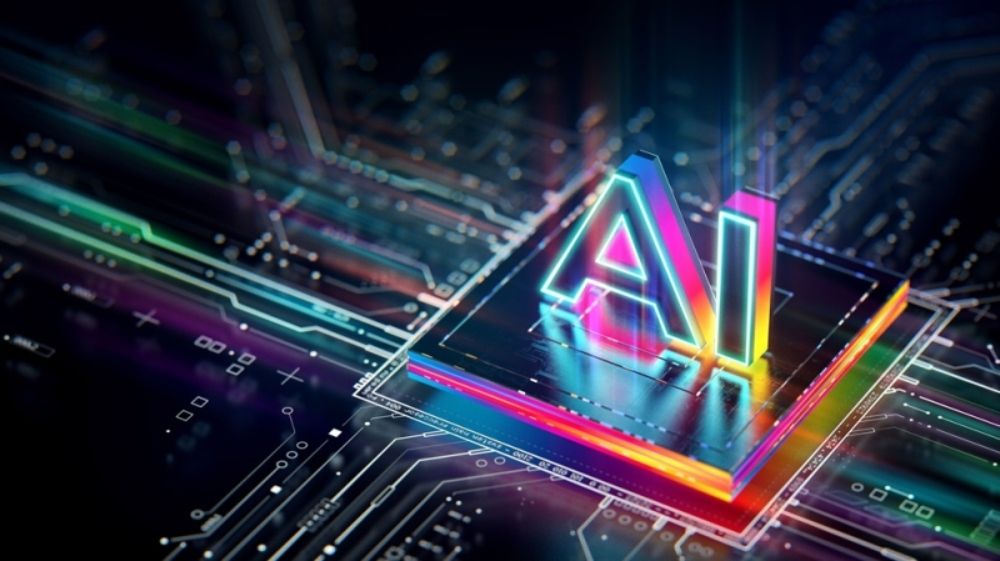
“Do we want to force every person in Southeast Asia to adapt to the machine, or do we want to make it more accessible so people in the region can make full use of the technology without having to be an English speaker?” he said.
“We are not trying to compete with the big LLMs; we are trying to complement them, so there can be better representation of us,” said Teo, senior director for AI products.
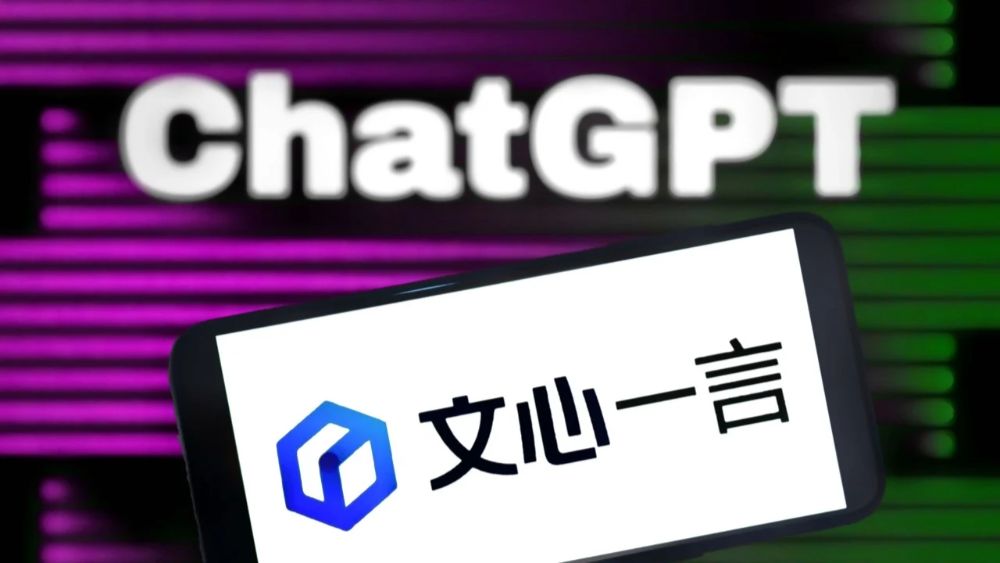
Despite there being over 7,000 languages spoken worldwide, large language models (LLMs) like OpenAI’s GPT-4 and Meta’s Llama 2, which are utilized to develop AI systems such as chatbots and other tools, have predominantly been created for and trained on the English language.
Governments and technology firms are actively working to address this disparity. For instance, India is creating datasets in local languages, the United Arab Emirates has developed an LLM powering generative AI tools in Arabic, and there are AI models in China, Japan, and Vietnam that operate in local languages.
According to Nuurrianti Jalli, an assistant professor at Oklahoma State University’s School of Communications, these models can play a crucial role in enabling local populations to more fairly participate in the global AI economy, which is currently largely dominated by big tech firms.
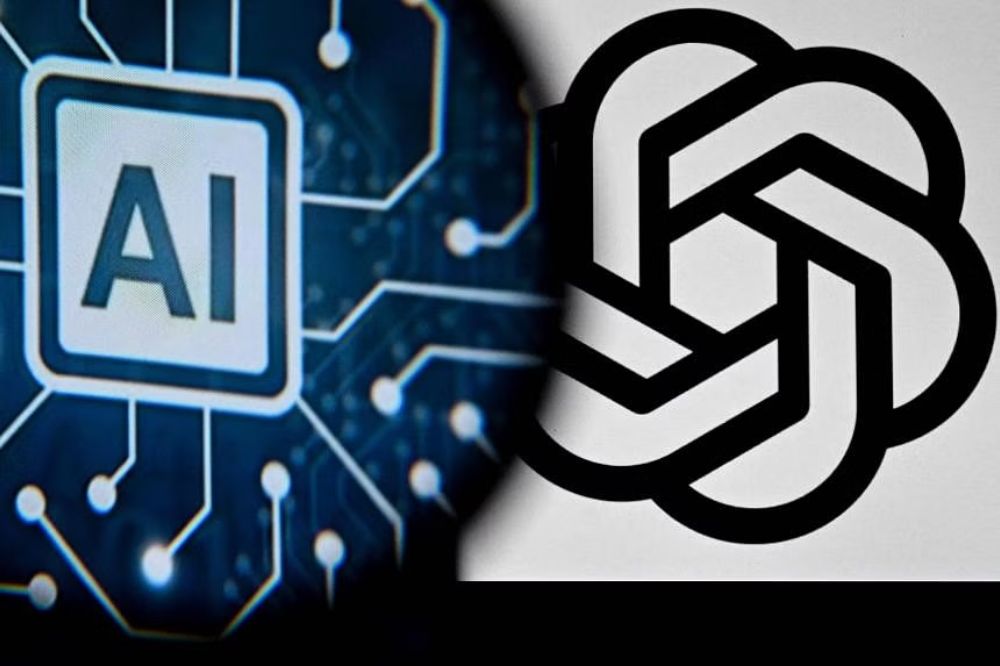
Researchers state that multilingual language models, which are trained on text from multiple languages simultaneously, have the capability to infer semantic and grammatical connections between high-resource languages with ample data and low-resource languages.
These models find applications across various fields, including translation, customer-service chatbots, and content moderation on social media platforms. They particularly aid in identifying hate speech in low-resource languages like Burmese or Amharic, where such detection has been challenging.
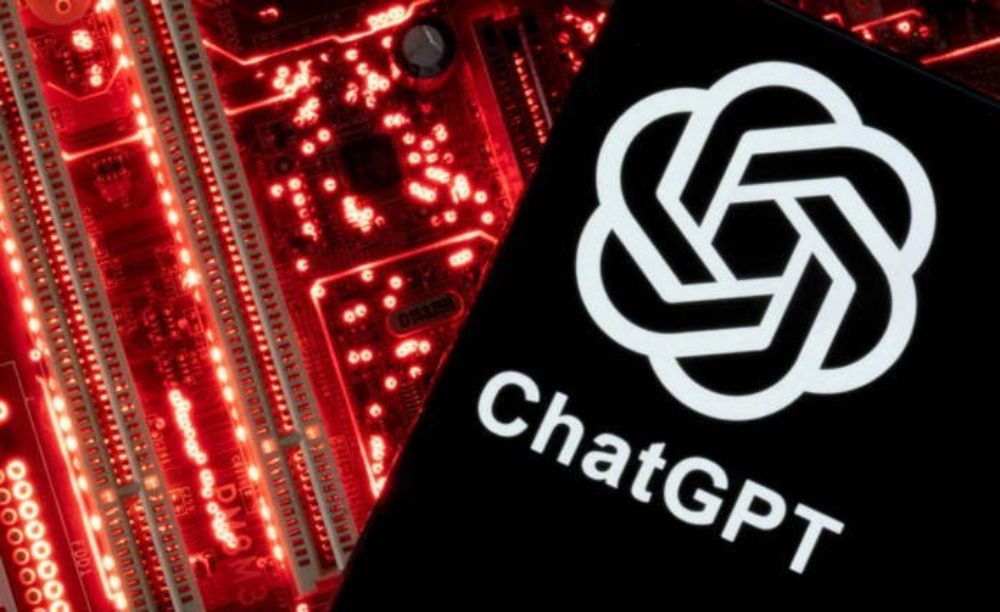
According to Teo, more than 13% of SEA-LION’s data originates from Southeast Asian languages, a higher proportion compared to other major multilingual language models. Additionally, over 9% of its data is sourced from Chinese text, while approximately 63% comes from English.
Given that multilingual language models often train on translated text and other data of varying quality, AI Singapore exercises caution in selecting the data used to train SEA-LION, as mentioned by Teo during an interview at the National University of Singapore.