In the realm of AI-powered chatbots and language models, one of the inherent limitations has always been their reliance on static, outdated data. When asked about the effectiveness of Covid vaccines against the latest variants in 2023, OpenAI’s ChatGPT admitted its incapacity, stating it lacked access to real-time information beyond its last update in September 2021. This deficiency highlights a critical challenge in the medical field where constant advancements and research breakthroughs are the norm rather than the exception.
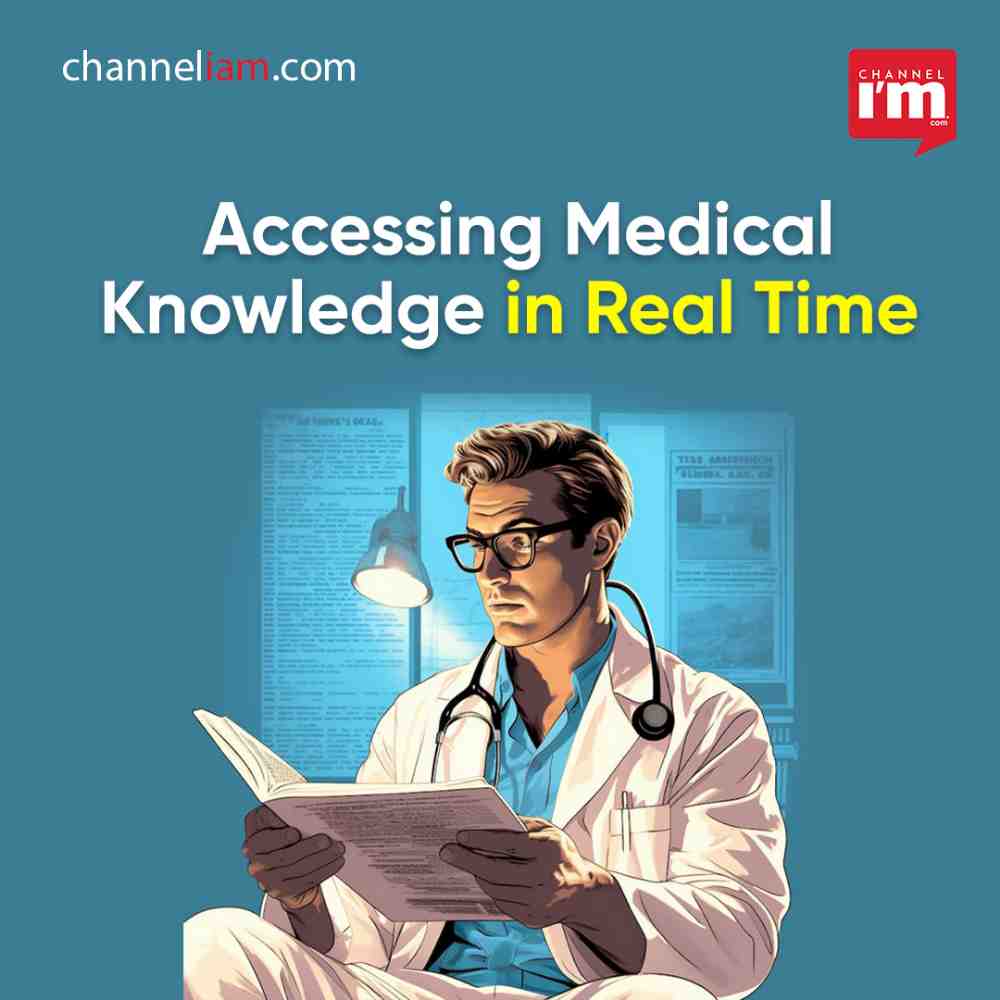
Daniel Nadler’s Ambitious Solution
Enter Daniel Nadler, a seasoned entrepreneur who previously sold his AI startup, Kensho Technologies, to S&P Global for a whopping $550 million in 2018. Witnessing the information overload faced by healthcare providers during the Covid-19 pandemic, Nadler recognized the pressing need for a tool that could separate valuable medical insights from the noise. He saw a parallel with his experiences in the world of finance, where AI was used to identify arbitrage opportunities within massive datasets.
The Birth of OpenEvidence
In November 2021, Nadler founded OpenEvidence, a startup with a mission to revolutionize access to medical knowledge. Armed with $32 million in capital, a team of nearly a dozen experts with PhDs, and a powerful supercomputer in the Nevada desert, OpenEvidence set out to tackle the challenge of providing real-time, up-to-date medical information to professionals.
Retrieval Augmented Generation: Marrying Language Models with Real-Time Data
The solution, as Nadler puts it, involves “marrying these language models with a real-time firehose of clinical documents.” This technical feat, known as “retrieval augmented generation,” empowers AI chatbots with the ability to access the latest research and clinical data right before answering a question. In practical terms, it means that when asked about vaccines and new Covid variants, OpenEvidence’s chatbot can respond with information from studies published in February and May 2023, complete with citations.
Quality Matters: Separating Signal from Noise
One of the challenges in dealing with vast amounts of data is determining the quality of the information. OpenEvidence takes this into account by considering the impact factor, a measure of a journal’s influence in the scientific community, when retrieving information from journal articles. This ensures that the AI’s responses are based on credible sources, enhancing the reliability of the answers.
The Interactive Advantage
Unlike static pages of text, OpenEvidence’s AI is interactive. This means users, typically medical professionals, can tailor their questions to specific patient scenarios, ask follow-up questions, and receive precise, context-aware answers. It can scan through tens of thousands of journals, providing a more comprehensive knowledge base compared to traditional sources.
Overcoming Challenges and Ethical Considerations
While retrieval augmented systems like OpenEvidence can reduce errors, they are not infallible. Just as humans can make mistakes when sifting through information, so can AI. The technology’s use is also regulated and guided by ethical considerations, ensuring it enhances medical professionals’ capabilities rather than replacing human judgment.
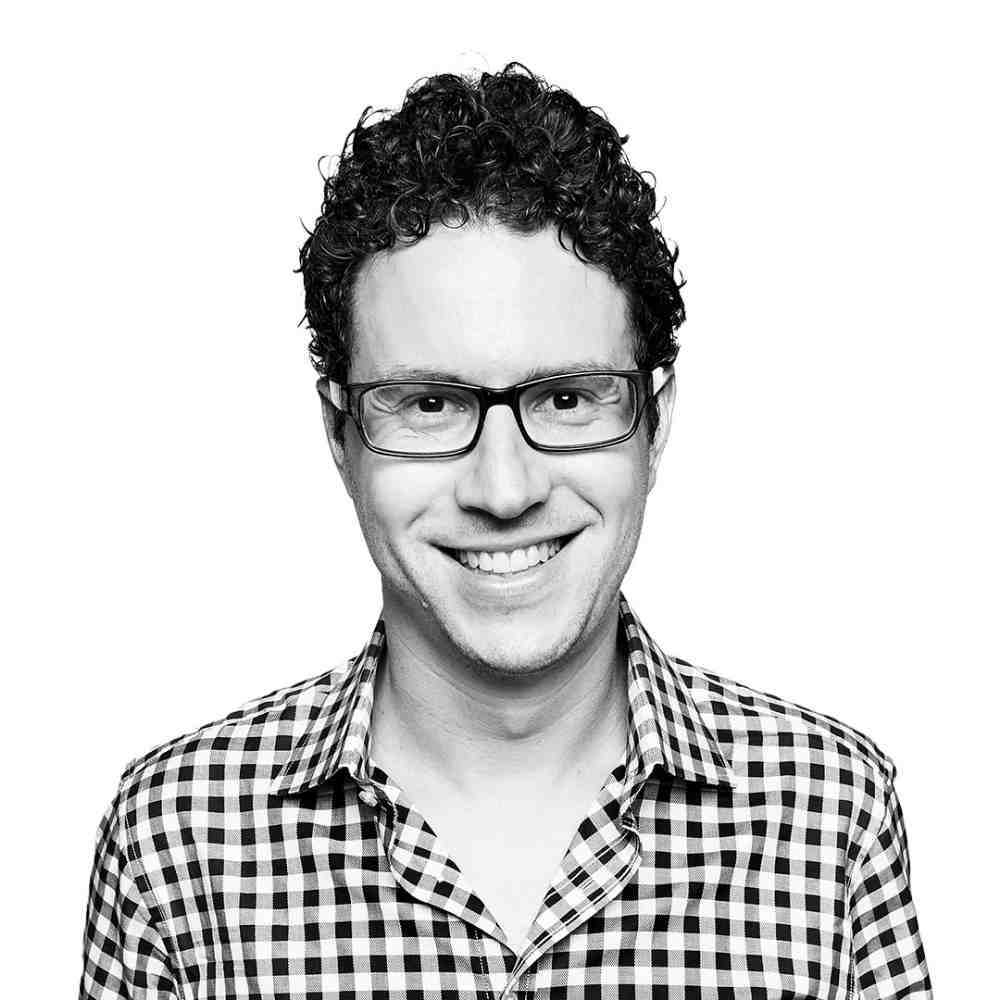
The Road Ahead for OpenEvidence
Currently free for licensed medical professionals, OpenEvidence is considering revenue models, including subscriptions and advertising. However, the startup’s primary focus remains on aiding healthcare workers and improving patient outcomes by providing them with the latest medical knowledge at their fingertips. As Nadler aptly puts it, OpenEvidence is not looking to become a chatbot for the average patient but rather a tool to empower medical professionals.
In a world where information is abundant but time is scarce, OpenEvidence’s innovative approach promises to bridge the gap between cutting-edge research and medical practice, ultimately leading to better healthcare outcomes.